The Online Master’s in Data Science
The world runs on data, and the leaders who understand its tools and techniques will win the race to the top. The Online Data Science, M.S. from New York Institute of Technology is made especially for those leaders—those who see data for what it is: an opportunity.
Push your expertise further in data science concepts and methods to reach the next level of your career. Armed with the latest skills and a degree from a top 15 university in the region, there’s no telling where you can go next.1
Program Details:
- 30 credits
- 100% online
- Complete in as few as 10 months*
- No GRE or tech prereqs required to start
- 3 opportunities to start per year
*Completion time may be as short as 10 months if previous education satisfies the prerequisites. Talk to an admissions outreach advisor to see if you are eligible.
Drive Your Career Forward
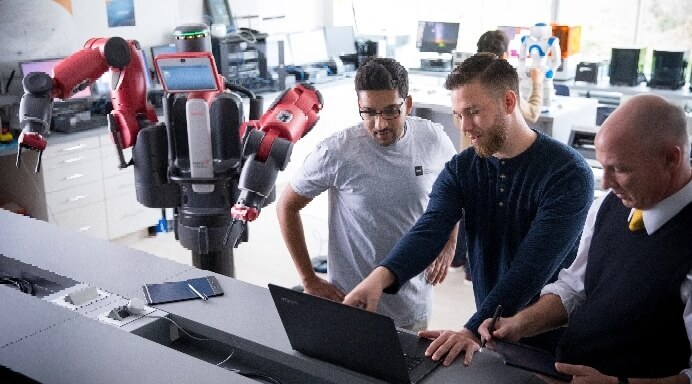
Put Yourself in Demand
Lead in one of the fastest-growing, well-paid positions today
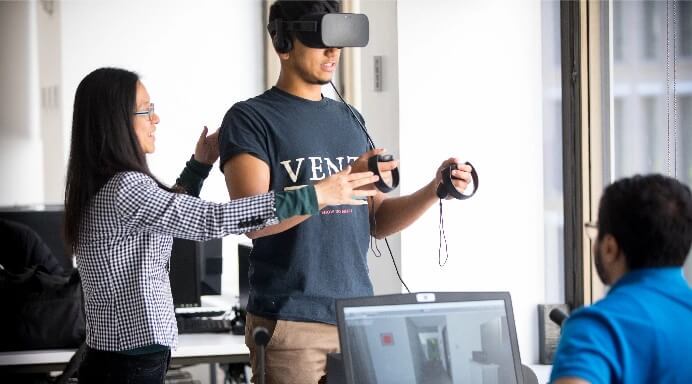
Make Your Learning Lucrative
Top 10% of U.S. Colleges for Return on Investment2
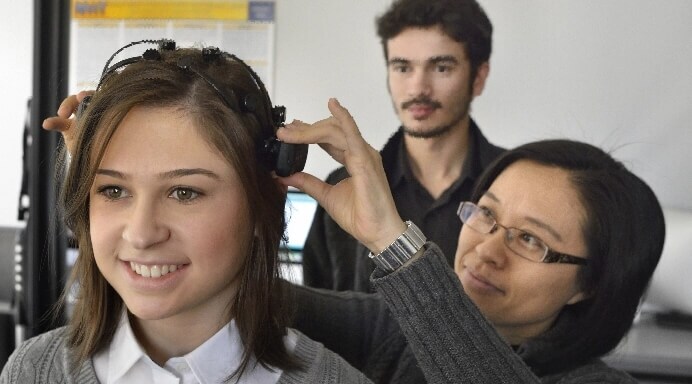
Explore Your Options
Apply your skills in healthcare, finance, biotech, and more
Master Emerging Tech
Considering a Master of Science (M.S.) in Data Science to take your career to the next level? Your journey starts here. Complete the form to get a program brochure for New York Tech’s College of Engineering and Computing Sciences online program.
Get valuable insights into the online experience, learn more about the College of Engineering and Computing Sciences, and see where this degree can take you.
This will only take a moment.
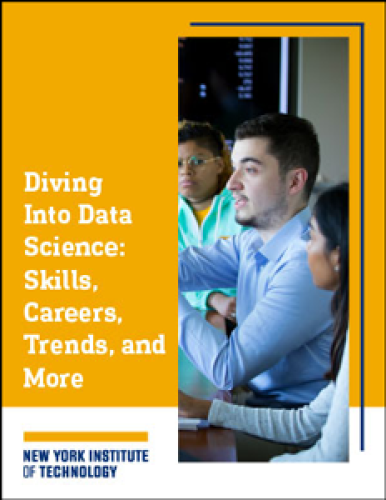
The E-book from New York Tech
Looking to pivot into data science or advance your career in tech? Get ahead with our free, comprehensive e-book that explores careers, essential skills, and more.
Download Now
Essential Data Skills for Dynamic Careers
It’s no secret that modern business is defined by data. At New York Tech, you’ll learn both the cutting-edge and practice-oriented aspects of data science to tackle any situation. When you commit to furthering your education in data science and its related fields, you’ll become highly valuable in the job market for years to come.
Program Takeaways:
- Computing theory
- Advanced data science
- Machine learning
- Data visualization
- Statistics
- Programming languages (SQL, R, SAS, Python, etc.)
Find Your Niche
At the end of the Online Master’s in Data Science program, you can choose to complete a thesis as part of your degree. Whichever subject matter you choose, the in-depth research and experience you complete will help you gain specialized skills and knowledge to better qualify you for research and development jobs. You also will have the chance to work on funded research projects with faculty members from sponsors such as the U.S. Department of Defense, National Science Foundation, and National Institutes of Health.
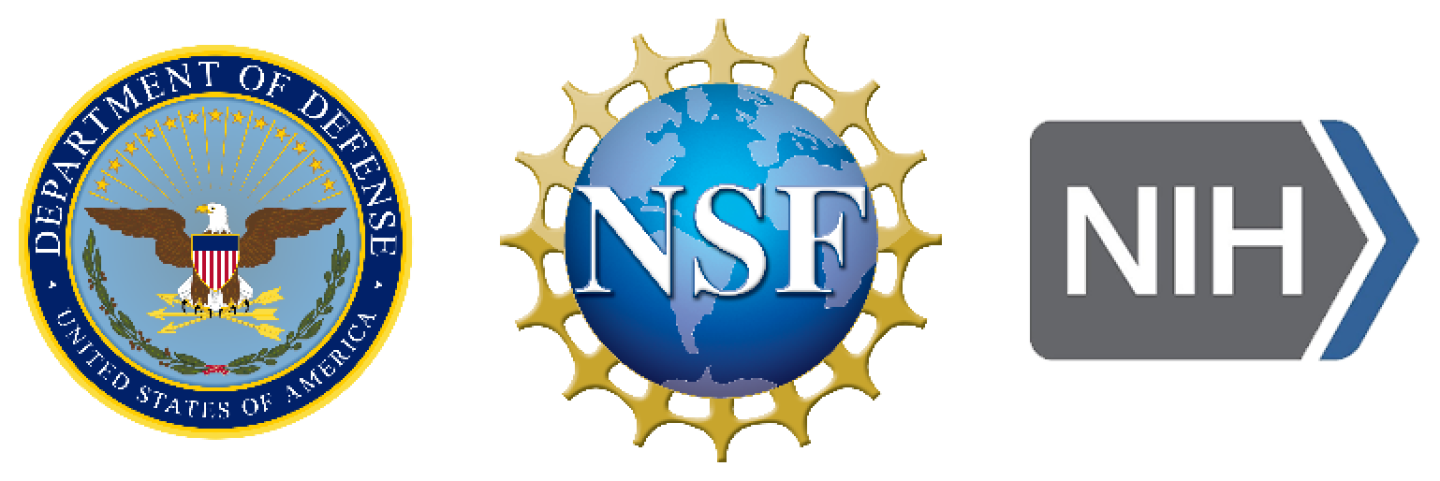
Get the Executive Perspective on Data Science
Two expert tech leaders offer real-world insights and frank advice for you as you consider your future in data science in this recent webinar, “Transition Into Tech: Unlocking Opportunities With a Data Science Degree.”
Sanyogita Shamsunder, chief operations officer for the technology company NextNav, and Barbara Porter, chief operating officer and chief technology officer at the online retailer Fragrance.com, answer commonly asked questions about data science careers and how to prepare for them.
They also share insights into their journeys from aspiring female students to the C-suite and highlight the many career options for master's-level data scientists. Watch the video or read the transcript to gain valuable insights for your career.
Video Transcript
Aisha Rudd: All right. So my name again is Aisha Rudd. I want to just go over the agenda. We're going to do some admissions team introductions; also our guest speakers as well. Talk a little bit about New York Institute of Technology, the online data science master's program overview. And then we're going to jump right into our Q&A after our panelist introductions as well.
Aisha Rudd: Ready whenever you are. Jeffrey, thank you so much. So myself again, I am Aisha Rudd. I am with Everspring on behalf of New York Institute of Technology. I'm the admission outreach advisor. I work primarily with the most critical part, in my opinion, which is getting started with your program. So, learning the ropes of the university, where to go for your documentation and things of that nature, and also any questions. If you're not sure who to go to, you can always give me a call.
Aisha Rudd: So I look forward to connecting with anyone that is live with us now and also in the future to assist. I'll pass it over to Jeffrey Bania, who is the director of admissions outreach for introductions. Here you go. Take it away, Jeffrey.
Jeffrey Bania: Yeah, absolutely. Thank you so much, Aisha. My name is Jeffrey Bania, director of admissions outreach. And I work very closely with Aisha throughout the beginning stages of someone's journey. So, we're really here to partner with you all when you're just exploring New York Tech and the data science master's program offered in the online format. Just to make sure that it's a great match and fit for what you're looking to study at the graduate level, and also to lead you to your future career goals and higher educational goals. So, thank you so much for being here.
Jeffrey Bania: I don't want to spend too much more time on my role and Aisha's because we have some very incredible guest speakers here with us tonight that I would like to provide a brief introduction before passing it over to both of them. They are both near and dear members of the New York Tech community. First, we'll begin with Barbara Porter. So Barbara is the chief technology officer and chief operating officer at fragrancenet.com and has been with the organization since 2010. In both her roles, she oversees all operations and technology, including business intelligence and data engineering or machine learning functions.
Jeffrey Bania: Prior to fragrancenet.com, Barbara served in several roles at 1-800-flowers.com in web development, customer application development, and enterprise initiatives. Before beginning her professional career, Barbara earned her Bachelor of Science in computer science with a minor in electrical engineering from New York Tech. Barbara also holds an MBA from the University of Massachusetts Amherst and an MS in cybersecurity from New York University. Barbara is a proud advisory board member for the College of Engineering and Computing Sciences at New York Tech, and we are so, so excited to have you with us here today. Barbara, do you mind adding anything else and welcoming everyone here?
Barbara Porter: No, I thank everyone for joining us today. I did want to point out that both of my master's degrees were through online programs. So it's a format that I'm very familiar with. And I just earned the second master's but my MBA, I earned 20 years ago, almost. So I do credit that for really helping my career move along to the next level while allowing me to function in industry and progress my career and my education at the same time.
Jeffrey Bania: Thank you, Barbara. That's fantastic. And a really great point that you mentioned there as well, especially since many people here today are considering that online flexible format so they can keep on working. Also with us here today, Dr. Sanyogita Shamsunder currently serves as the Chief Operating Officer at NextNav. In addition to NextNav, Sanyogita has over two decades of leadership experience at major tech companies like Verizon and Google, where she led global teams.
Jeffrey Bania: In her roles, she has spearheaded 5G technology, network strategy, and Google Cloud's edge networking. She has also advised startups on product roadmaps and go-to-market strategies as well. Sanyogita earned her MBA from the Wharton School at the University of Pennsylvania and a PhD in statistical signal processing and math — which I can't even fathom to think of what that degree plan looks like — from the University of Virginia. Sanyogita is a proud advisory board member for the College of Engineering and Computing Sciences at New York Tech. Welcome, Sanyogita. Would you like to add anything else as well to all of our attendees here today?
Sanyogita Shamsunder: No, thank you for inviting me to this exciting panel. I'm looking forward to interacting with the potential students of this online program. So let's get going, I guess.
Jeffrey Bania: Absolutely. Without further ado, we have some great questions to get through. I think, Aisha, you did want to go over some points about New York Tech as well before we get into those questions and the Q&A feature, all of that. So back over to you, Aisha, and I'll be sure to share some helpful links in the chat should anything come up.
Aisha Rudd: Thank you, Jeffrey, and thank you, Barbara, and also Sanyogita for being here; as well as everyone else that's here. I'll go over a bit of an overview about New York Institute of Technology, and then I'm so excited to jump into the Q&A. We have so much experience in this room today, and I am looking forward to the discussion. First, just a few little highlights about New York Institute of Technology. This is a private, non-profit university that offers over 100 highly ranked, accredited, and in-demand degrees in areas of specialization. I have sat in a bunch of meetings and am learning a lot more that New York Tech is becoming the tech hub of the world, so great opportunity. The network is boasting over 110,000 alumni, and as you guys can see, as everyone here can see from some of the rankings here, Top-50 performer in social mobility for regional universities in the north, and also a few other accolades that you see there.
Aisha Rudd: So if you found New York Institute of Technology, you are doing something right. So ready when you are, JB, to give a brief overview of the program. The online data science master's program: This is a program that I am the admission outreach advisor for; connect with pretty much everyone that is interested in this journey with your master's degree. It is 100% online. You have an opportunity to graduate in as few as 10 months. That is not the norm. On average, I would say this is roughly about a year and a half program, but there are a lot of opportunities for you to fast-track this even quicker, depending on what your goals are. There are three opportunities to start per year. There is a spring, summer, and fall semester and a lot of opportunities for access to on-campus resources like the Entrepreneurship and Technology Innovation Center. Also, you do not have to have any prior tech experience to apply for the program. I think that is a very good point that not many other universities are doing. So New York Tech is definitely doing something right.
Aisha Rudd: They have prerequisites that are part of the program that will help eligible students move further along through their master's journey. So very excited about that. Also, if you notice, Jeffrey is putting in the chat here some very helpful links for anyone. I would definitely recommend saving those. And also, we are going to have Q&A at the end. So you should see a Q&A icon at the bottom. If you do have questions, please feel free throughout the conversation to go ahead and put those in the chat and we will make sure that we take care of those towards the end. So thank you so much. And we're going to get started here. So today's special topic is “Transition into Tech: Unlocking Opportunities With a Data Science Degree.” So, awesome experience. As I mentioned earlier, there's a lot of experience in here. So I'm excited to dive into the questions here. So the first one we have for our panelists, “What data-related skills do you look for when hiring candidates in your respective organizations?”
Sanyogita Shamsunder: I can go first if you want.
Aisha Rudd: Yeah, sure thing.
Sanyogita Shamsunder: Yeah. So it really depends on the teams that you're going to be joining. So it's a combination of data science skills, as well as this domain expertise. Let's say you are joining an HR organization. What you need in terms of data-related skills may be a little bit different than what you might need if you're joining an engineering or software development organization.
Sanyogita Shamsunder: But the bottom line is to be able to manipulate data, work with data, do some basic analysis from a statistics and analytical perspective, being able to explain the data, ask the right questions of the data. What problem are you trying to solve? Or, what are you looking for in this data?
Sanyogita Shamsunder: If you're joining an HR organization, it may be how long did it take for this position to be filled? Or, how long did this particular employee stay in the organization? And what were some of the things that maybe impacted this individual's performance? Or, why did they leave? Why was there attrition? So being able to ask those questions is also extremely important. So it's a combination.
Aisha Rudd: Awesome. Thank you for that. Barbara, did you have anything you would like to share as well?
Barbara Porter: Yeah, sure. So, definitely any tech-related field, including data science, I look for candidates who show the ability to learn and evolve. The tools and the techniques that are being used, they'll be different in three years, in five years. So you need to be willing to continue your learning, and not necessarily in a formal environment, but learn how other data scientists are solving problems and adapting to the changing tools and techniques. I think that's important.
Barbara Porter: You should enjoy analyzing situations and problem-solving. So trying to understand what the data is telling you, telling your company, and helping to form solutions, or how it can drive outcomes in the actions that a company takes. I think that's something that you need to feel comfortable with. And understanding of statistical concepts, certainly. SQL. So in my organization, our data scientists are working directly in the database. They're writing ad hoc queries. So being able to write SQL. And if you don't know what SQL is now, or you don't know how to write SQL queries now, don't worry. You will learn in the program. But these are important ways of being able to get the data you need, and then you can analyze it from there.
Barbara Porter: Python is becoming more and more prevalent with data scientists, especially in the machine learning realm. And it's not hard. And even if you've never programmed before, it's actually one of the easiest languages to learn. And I know I personally took a machine learning class a year ago and was surprised. I haven't actively programmed in many years. And I picked up a little bit of Python, and it was more than sufficient for what I need.
Barbara Porter: There's a lot of tools out there, especially with machine learning, where you only need to write a few lines of code to be able to build a machine-learning tool for yourself. It's really about learning what data to include and how to prepare that data to be consumed by a machine-learning tool. So the actual coding piece of it, do not let it scare you. It's not overwhelming. It's something that you could actually pick up on your own. But I believe the school includes that in the program as well.
Sanyogita Shamsunder: Yeah, those are some good points Barbara made. Bottom line is curiosity and some data background in terms of using Excel, being able to identify what type of data is important in a given situation, what to include and what not to include, and how to present, eventually, what you get. What questions to ask. The coding part, yeah, I mean, I think it has gotten a lot easier with the languages that are available. And of course, there are a lot of no-code, low-code platforms that are available. So that shouldn't be a big barrier these days.
Aisha Rudd: Thank you. That was a great question. A lot of candidates that I connect with — that are interested in this program — those are some of the highlights, programming languages, machine learning, Python, things of that nature. So appreciate you Barbara, Sanyogita for being very descriptive with your answers with this. I think it goes a long way, so thank you. We'll move on to the next question. “What role does data science play in your respective industries? What do data scientists focus on in your respective organizations?” And I think, if you don't mind, Barbara, we could start this one off with you, if you're okay to start this one off.
Barbara Porter: Absolutely. Our data scientists are looking at all of our sales figures. So understanding, first of all, they're very involved in forecasting what we anticipate our sales will be, but then they analyze what our sales have been to see if their forecasts are accurate. The forecasts, I work in e-commerce, we sell fragrances. If our forecasts are wildly off, we're going to buy too much or too little inventory. And neither one of those is a good situation for the company.
Barbara Porter: So, we really rely on good forecasting. And part of that is understanding when our sales trends differ from the forecast, really digging into why that could be. What are the reasons for that? Could it be because marketing didn't run a promotion that they anticipated? Could it be that what a recession has hit? And nine months ago when we did a forecast, we didn't anticipate that recession. Things like that. There's always usually a reason you can attribute it to if it's a significant swing. So that's very important.
Barbara Porter: Consumer preferences, those change over time. So yes, we're fragrance net, but we've actually branched out into haircare and makeup and skincare and kind of related beauty items. And we've done that because we saw consumer tastes were shifting and our customers were interested in those other related items. So we added those to our catalog. As I'm also chief operating officer, I'm over our warehouse operations. So I'm always looking at how well our warehouses are functioning, how much effort it takes to get an order shipped and looking for ways to remove friction from that process. And in an ideal world, we get goods in one door and we're shipping them out to the customer through the other door. But that's not the reality.
Barbara Porter: Sometimes you have too many goods, you put them on the shelf, you wait for those orders to come in. But then how do you pick those orders efficiently when you're dealing with warehouses that are the size of a football field and may have 60,000 to 100,000 different locations you can pick pieces from. So it gets very complicated. And we use that data to help find and optimize the process — find efficiencies, optimize the process — to make everything just flow as smoothly as possible. So those are just some areas we focus on.
Aisha Rudd: That's great feedback right there, thank you. Sanyogita, did you have anything to share for this question?
Sanyogita Shamsunder: Yes, I come from a very different industry. Barbara had some very good examples that we all identify with on the day-to-day basis. But I come from, I spent a lot of time in the telecom, wireless, mobile and networking industry. So data is the fundamental thing that we use to run the network. For example, there are some issues in the network. We have different, what we call probes in the network to be able to gather data and see how a particular cell site is doing, for example. Or inside your cell phone, there's a whole lot of data analysis going on all the time.
Sanyogita Shamsunder: So it's a very deep-tech type of data analysis on one side. So that's one end of the spectrum. And you use that data also to figure out where to deploy the next cell site so that you and me as consumers get better coverage, understand where the calls are dropping, for example. So that's one part. On the other part though, there's customer experience, customer calls. How long did it take for us to respond to a customer call, for example. How long did this issue last? And how long did the rep spend time? How many minutes of the rep's time was spent in resolving a customer issue? You wanna be able to reduce that or make sure that your customers are getting the right help quickly.
Sanyogita Shamsunder: So that's one end. And of course, again, I'm the chief operating officer at NextNav and my company does a lot of deep tech. So we do position, location, and timing. And what we are building is a backup for GPS systems. GPS is what we use. For example, everything that we do today runs on location. We are constantly using it without knowing it, whether our packages are getting delivered or we are looking at navigation, supply chain tracking, everything depends on location. So we are building a network that kind of creates a backup for this. And one of the things that you have to understand is the GPS system going down. For example, when there were solar flares, GPS was impacted in some areas. That requires us to collect data and analyze the data to understand if there was an impact to GPS, because that eventually impacts the economy, as well. So that's one part.
Sanyogita Shamsunder: And then similar to what Barbara said, how can we improve processes? How long did this project take? Could we have done better? Doing analysis on that, making sure that we are using the right tools to track some of the projects and data is a big part of that.
Sanyogita Shamsunder: Going to, again, HR systems, right? Attrition, why did this employee leave? Being able to understand that, like collecting data from different sources and understanding impact of, let's say, particular policy on employee performance. That requires data analysis. So regardless of which area of the business that you're looking for, data is a fundamental part of running the business in a better way. And all parts of the business have their own metrics to track and use data as part of that.
Aisha Rudd: Well said, you both. Thank you so much for that. Let's go on to the next question here. Awesome. Thank you. So next question up we have, “What are some misconceptions of careers within tech or big data?” I think I like going back and forth. So Sanyogita, if you don't mind, can you lead us with this question if you have anything to share for this one for us?
Sanyogita Shamsunder: Yeah, I think one of the big misconceptions is that they said data science is a new field and that it's for fresh grads. That's not really true. Data science has been around for a long time. Maybe we didn't call it data science. Like my PhD in statistical signal processing, that's a lot of data analysis. So we've been using data, always. As a discipline, it has kind of become a little bit more maybe structured, now. So it's for everybody.
Sanyogita Shamsunder: And it's extremely important to have domain knowledge, right? It's coming from, let's say a neuroscience background to be able to understand how the brain works, collecting data, EEG data, running it and understanding underlying phenomena. That could be data science. I mean, that also involves data science. And it's not just... And the other misconception is that you are sitting in a corner by yourselves and programming all the time. That's not true. All the examples that I gave you require you to have more broader experience and knowledge than just programming.
Sanyogita Shamsunder: Of course, there are jobs that just require programming, no doubt about it, right? That's what they do. That's what that job needs. Like if you're developing algorithms for analysis, machine learning and all that, that's very techie. But it's a wide spectrum of skill sets and, hence, career types. So your understanding of the domain is extremely important. Being able to talk to others and understand how they're collecting the data that you need to process is important, right? Giving them requirements on what do you need, right? And how do you visualize, present, Ask the right questions, I think. So those are a couple of examples. I don't know, Barbara.
Barbara Porter: Yeah, I agree with what you said. I don't wanna scare anyone with... You've mentioned like not having an understanding of neuroscience if you're working with that kind of data. Absolutely. I will tell you, before I took an operational role here or as I was getting more involved with operations, I looked back to when I was in college many, many years ago. I worked part-time in a grocery store and I saw how they stocked the shelves at night and managed their inventory and things like that. And those concepts actually carried with me into this role as I got more involved in operations here. There's some similarities here.
Barbara Porter: So regardless of your current background or experiences, there's a good chance that there's a company out there. Listen, every company has data and should be leveraging that data to its own benefit to help the company grow and thrive. And if you have some sort of relevant experiences, you could be very valuable to that company. As Sanyogita said, you're not just stuck in a corner all day. You're not just stuck behind a keyboard programming all day. In our company, the data scientists are involved in all of our meetings to understand what kind of data we have, what kind of data we could collect. Obviously, there are issues around privacy and what you do with data, and personally identifiable data. So those are things we need to be aware of.
Barbara Porter: But they're a participant in these discussions on how to grow the business by leveraging our own data. So if there's data they feel that would be helpful, that we might be able to collect, they wanna voice that. They're helping us grow this company, so they are not just on the sidelines reporting on what happens. They're actually part of how we're moving forward as an organization.
Aisha Rudd: That's awesome. Thank you both for sharing. As I'm listening to how you ladies are answering the questions, it does present a very realistic day-to-day operations for data science versus it being so technical. So I definitely do appreciate that. It really does help with the experience that you're both bringing from different industries. So thank you so much for that. I’m really enjoying this. Let's go on to the next question here. So thank you, Jeffrey, for that. “How does data inform your day-to-day work?” This is a great question. And Barbara, if you don't mind, did you wanna start us off with this one?
Barbara Porter: Yeah, so as Chief Operating Officer, I see almost every department in the company. And when I see a possible issue or possible dysfunction or inefficiency in the organization, it could be related to cost. If I see costs growing unexpectedly, customer satisfaction either increasing — which would be great — or if it decreases, that's a concern we want to address. If our profit is changing, things like that, we wanna know what's driving it so that we can find ways to either do more of what's working well or do less of what's not working so well.
Barbara Porter: So there's not a day that goes by that I don't work with our data scientists and kind of pose questions to them. I will explain that I see something in this area. Can they dig into that a little bit further and tell me what they believe is going on and give me some perspective there? So they are really, they're kind of, when I see an issue, they're the ones who go out, they explore the data and they come back and they tell me what they see and help us figure out what changes we should look at that might improve things. So they are definitely very valuable to me in my role. So that's my answer for that.
Aisha Rudd: Awesome, thank you so much for that, Barbara. Anything you would like to add to this question, Sanyogita?
Sanyogita Shamsunder: No, I think Barbara answered it, and it's similar, although our business is not directly to consumers. So some of the data analysis and gathering that we have is not necessarily related to customer service from a consumer perspective, but nevertheless, we are constantly monitoring our systems to make sure we are, let's say, so data is a part of it, of course, whether it's giving the right answers, like if it's meeting the requirements, are we driving the SLAs? Are we meeting the SLAs that we promised to our customers? So all that requires data analysis on a regular basis. It's almost a part of doing business. And then, if there are things going wrong, identify the root causes and for that, you need to collect more data and analyze it to see what went wrong in a particular scenario. So in our case, some of these things are inherently a part of the job in many of the teams.
Aisha Rudd: Thank you. Thank you for that. Appreciate that. And let's see what our next question is. So, “What is one common misunderstanding about the role of data science in your field?” And Sanyogita, can we start this one with you, if you don't mind?
Sanyogita Shamsunder: I think we kind of covered it in the previous question. So primarily, I think that …hat a data scientist is somebody who's like sitting and programming all day, right? I think that's one misunderstanding or misconception. And that data is, there's like privacy issues regarding, I mean, do you always have access to data? Maybe not. So you have to be creative about how you collect the data without kind of violating some of the rules in terms of privacy, especially when you're dealing with consumers. I think that's extremely important. And that data never comes very clean. There is always issues. And that's one of the big parts of a data science, maybe understanding the course, the program that we're talking about is to be able to understand how to basically manipulate the data or clean the data to make it useful for further analysis. I think that's the other part. Like it's never that straightforward.
[laughter]
Aisha Rudd: I'm glad you shared that.
Barbara Porter: I'm sorry Aisha.
Aisha Rudd: No, go right ahead, Barbara. I'm sorry.
Barbara Porter: Yeah, I think the common misconception is that it's all about sales reporting. So how many orders did we take yesterday? How much money did we collect? That's reporting and that's not necessarily, that's actually not data science. I love, and I touched on this a little bit earlier, I love giving our data scientists kind of abstract problems and give them the freedom to figure out the right way to solve it. So, or to answer the question.
Barbara Porter: So for example, we currently have three warehouses around the United States. We only opened the second one two years ago. So going back about four years, we had a warehouse in New York and we had a warehouse in Nevada. And we posed the question to our data science team, we wanna open a third warehouse. What's the best location to open that warehouse in? So they had to look at various factors. Where are our customers? What's the heat map? If you look at the United States, what's the heat map of our customers? If you have no customers, in a certain area, then you shouldn't really be putting a warehouse there because, you're not gonna gain any efficiencies. What are the shipping costs from various areas to other areas where your customers are? So Lexington, Kentucky is big for a big hub for UPS. Memphis, Tennessee is a big hub for FedEx.
Barbara Porter: Does that factor into the thinking? What are labor rates? Sorry Sanyogita, but we all know that California is an expensive state to operate in. My company will likely never open a warehouse in California, right? So certainly that has to be a part of the equation, when you're looking at things like that. And then real estate, right? So what is the cost per square foot? So a good example, I mentioned that we're in Nevada, we're in Reno, Nevada, which is not terribly far from where Sanyogita is. The labor rates and the real estate rates are drastically different between where she is and where our warehouse in Nevada is.
Barbara Porter: So all of those things got factored in. I didn't give them these things to look at. I just said, where should we put our next warehouse? And they looked at all of those things and they came up with a few areas in the Southeast. We ended up choosing an area in suburban Atlanta.
Barbara Porter: But honestly, we're starting to think about our fourth warehouse. And I'm gonna be asking that question again, but those are the kind of, you're not just being told go add one plus one and tell me what the answer is. It's not a very boring, dry job. You actually can get — if you're working in a good company — you'll be given the creative freedom to come up with unique perspectives on the data and what to do with it.
Aisha Rudd: That was a great question. I love those answers. Thank you. Thank you for that. Let's see what we have up next. That was awesome. All right. So, “What challenges have you faced as women in your respective industries?” Another great question. And I'll let you ladies, whoever wants to begin this one, you can go ahead and chime in.
Barbara Porter: I'll go for it.
Aisha Rudd: Awesome.
Barbara Porter: So I started my career a long time ago. I went to college and had a lot of fun, and didn't get a degree right away. So I found myself in the workforce. I was an entry-level bookkeeping clerk for a petroleum company, not a particularly interesting job or sexy job. But I realized pretty quickly, here I am 19 and I'm working with — hate to say this now, 'cause I'm one of them —
but I'm working with a bunch of middle-aged women and realizing that that's what I was gonna be when I got to that age, unless I changed things. So that's when I got serious about school. I got myself into school at night, and really took things seriously. So I didn't actually get my degree and change into technology until I was 31. So I have a number of years of experience, working in not the most glamorous field, and women were not respected in that. We were kind of looked down upon because we weren't the ones who were making money for the company. We weren't doing anything with strategy. We were just literally adding one plus one and telling them that it equaled two. So it was not a good feeling for, especially for knowing I could do so much more. But the position I was in, until I had that degree, I wasn't gonna be able to prove that. Nobody was gonna hear me. So anyway, I did move over to IT after I got my degree from New York Tech and it was a different world.
Barbara Porter: So IT is much more of a meritocracy. So it doesn't matter where you were born, what gender you were born as or identify as, or…it doesn't matter. If you have the ability and you can do the role, you will be respected for that. And I will say, our data science team, we've got people who have graduate degrees. We have people who don't even have the bachelor's degree and worked, similar to me, worked in other areas of business, but then got interested in data science and they were able to get their skillset up to a level where they work very well for us. So again, it's my feel on it as a woman is, IT and data, it's much more of a great equalizer. If you can get yourself the skills and get yourself the experience, it's a much more level playing field than some other departments, even today. That's my perspective on things.
Aisha Rudd: Thank you for that. Thank you for that. And Sanyogita, did you have anything you would like to add to this?
Sanyogita Shamsunder: Yeah, so I was one of six women out of a class of 60 when I entered engineering. So very small, like less than 10% of the class was women. And I continued to, and I've always been in engineering. So in fact, I did a little bit something opposite of what Barbara mentioned here. I continued on to go to grad school, and then being in deep tech all the time. And then at some point I decided that I wanted to get a broader perspective that the business part was also very interesting to me. Understanding technology sometimes is the least of the issues, right? There is a whole lot of things that make a business successful. And being able to have a broad view of that, and being able to appreciate that is important. So I went and got a degree in management and finance. Actually, again, focused a lot on data and so on.
Sanyogita Shamsunder: But nevertheless, what type of challenges did I face? Well, I mean, I think early in the industry as I was having my first child, the maternity leaves were very short, right? Like two weeks or something like that. So I think that has been a challenge. I don't know that it has —
it's changed quite a bit now, thankfully. But I remember going back to work within two weeks and thinking that I didn't want to be a disruption for the team, for the company. And I think that's important as a woman. Of course, if you want to have children and so on, have a supportive culture from an enterprise perspective. I think that's thankfully has changed quite a bit now, maternity leaves are longer. And I also urge the younger me, I would have said, you should take more time off if you need to, right? I don't think it's — the sky will not fall just because you're out on maternity leave. So that's one of the challenges that I mention all the time.
Sanyogita Shamsunder: Personally, I think also like in the early days, it would be difficult for me to speak up in large teams, team meetings. So I remember one of my mentors, she became a mentor later on. She said, "You need to speak up, you have very good points to make, don't be hesitant." So that was another thing, like again, maybe a personality issue, but a lot of women do have that lack of confidence. Sometimes it's taken as you wanna be different, you wanna be like, you wanna make sure that you're saying the right thing, you don't know and you kind of hold yourselves back. So that is something that I think you should be recognizing and proactively working towards overcoming that fear.
Aisha Rudd: Thank you, thank you. Those are some great, great, great responses. Appreciate that and look at where you are now. So that shows growth of this industry as well. So I'm excited, I've really enjoyed this conversation. Let's see what we have next. Next question up, “How can a master's degree in data science support a career in tech?” Another great question and let's see here, Barbara, would you like to start us off with this one?
Barbara Porter: Yeah, sure.
Aisha Rudd: Thank you.
Barbara Porter: So I think of it as this way, in companies you have accountants and you have CPAs, right? And the CPA knows the advanced rules and regulations. They maybe have more knowledge, more specialized knowledge. And I kind of see that, listen, a lot of people in companies right now know how to analyze data to a certain extent. Most people know how to use Excel and they can do some reporting and maybe some basic analysis but it's those data scientists who are able to use advanced tools and techniques that will answer bigger questions and help companies in a bigger way, whether it be strategically or identifying performance concerns, making improvement recommendations, things like that. So it's having that master's degree shows an employer that you've gone through this advanced training, you have advanced skills and knowledge and you can contribute in a more specialized way than just somebody who's good at Excel.
Sanyogita Shamsunder: Yeah. Yeah, the other thing to add, I mean, that was a great point Barbara made. The other thing to add is when you're going through a program like this, which is a data science program, perhaps with a lot of interdisciplinary students who have come from many different backgrounds, you learn to have a perspective, a broader perspective, learn from each other, understand how data is being used in different industries, perhaps projects that you do together that will enhance your skillsets as well as build intuition in some ways, right? I think those are some things that you cannot possibly get when you're like working by yourselves or trying to, learning on your own. I think this type of an environment is great for building that skillset.
Aisha Rudd: Awesome, thank you.
Sanyogita Shamsunder: And plus I always enjoy going back to school, so.
[laughter]
Aisha Rudd: Yeah, I'm learning.
Sanyogita Shamsunder: I'm biased.
[laughter]
Aisha Rudd: You're always learning. So much knowledge, so much knowledge. Thank you so much for those great, great points. Let's see here. Our next question is, “What advice do you have for folks who are looking to transition into a career in technology?” Let's see here. Sanyogita, you wanna start this one off?
Sanyogita Shamsunder: Yeah, sure. I think identify, first of all, technology is a big field. Even in technical companies, like I said, I own IT, HR, program management, and so on. So it really, again, you have to identify what is your domain area that you're interested in, right? You could be a pure tech, okay. You're developing the software, or you're building the hardware, the systems. So that's one area, but then you have all these other things. Barbara gave great examples of how she uses data science, I mean, to determine where the next location is, right?
Sanyogita Shamsunder: Requires a different type of skillset. Marketing, using technology in marketing, understanding human behavior, consumer behavior. So a background in psychology or something like that is also important. So I think you have to understand what is it that interests you in the technical field, right? And take it from there. And, of course, having additional skill sets, like being able to understand the data in that particular domain will only enhance your profile and in a positive way. So that's my advice, is what's your North Star? What do you wanna do?
Barbara Porter: I agree; there's so much variety in tech. So I got my computer science degree many moons ago. I've never spent a single day working as a programmer. I know how to program. I did it a lot earlier in my career, actually, before I even got into school and then, of course, in school, but I've never been paid a day in my life for being a programmer. So it's not all about programming, and that's the same for data science. It's not all about programming. There is some parts of data science where you don't necessarily need to program. The tools can do things for you. So there's a lot of variety there. I think, in addition to being more of a meritocracy and more of a level playing field, in my perspective, these kind of fields and data science being included, there is more of an opportunity for work-life balance.
Barbara Porter: So during COVID, obviously, we sent everybody home initially except for our warehouses, everyone to work from home. And now the other departments, accounting and marketing and customer service, they've returned to the office. Our IT department, our data science department, they are not in the office. They are 100% remote. And I think that's more common in the tech fields than it is in general in business. For perhaps somebody who's got children at home, young children at home, or maybe they're taking care of their parents at home. Again, working with technology can make it easier to have either a hybrid or remote work lifestyle, which can work for some people much better than a traditional in-office work style. You should have some sort of comfort level with math and statistics. We talked about that. Python, you should absolutely try it. If you haven't already, it's not hard. There's a lot of free tutorials out there on the web.
Barbara Porter: You can do a good amount with it in not too many lessons. So don't be scared of it. And transitioning to tech can be literally life-changing. So I mentioned, I'd still be an accounts receivable clerk at this age if I hadn't made that decision 30-plus years ago, that that was not the path I saw for myself. That's not how I saw my life evolving. So don't be afraid to make that step, but I would recommend that you try to find somebody who is working in data science and just learn a little bit more about what their experience has been. Not every tech career, and perhaps even including data science, it's not for everybody. So learn a little bit about it, but it can be quite a rewarding career.
Aisha Rudd: Awesome, thank you so much. Thank you so much. I'm sorry, I couldn't hit mute quick enough. I'm taking notes here. Thank you guys. Thank you. This has been great. We'll field any questions that we may have that's coming in. I think I see one question. Hi, Alicia. Let's see here: A question for Alicia here. “I would love to hear from both ladies about their journey to get to where they are now. Did you struggle in the beginning of your degree? How did you overcome those struggles? Did you struggle in learning what your teachers were teaching? How did you overcome that?” Hopefully these questions make sense. Yes, they definitely do. And we'll open that up for the panel who wants to jump in on that one.
Sanyogita Shamsunder: Yeah, I can take that. Like I said, I was in a class with very few females and some of the early classes like physics. I always liked math, but physics was something that I didn't really enjoy as much. So that was hard. The way I tried to get over some of the challenges was from groups, study groups, talk to people, try to push through and saying this too shall pass.
Sanyogita Shamsunder: It was really by the time I got to second year or third year, that's when I started enjoying the classes. So initially it was a little bit of an uphill battle, but I did it by getting help from other friends in the class, study groups, study sessions and so on. So I would suggest doing that. I still advise youngsters in college, study groups are the best way to learn. You learn so much when somebody else is asking a question and maybe that you didn't think about. Those are great ways to learn.
Barbara Porter: Yeah, I'll add to that. So as I mentioned, my two master's degrees were both online and in both programs, my cohort, we created study groups, virtual study groups to support each other as we went through the different classes on the journey. So I've done it in a physical classroom setting, but I've also done it in a virtual setting like this online program. So you're not gonna be the only one that has challenges. And if you find good people who are as driven as you are to succeed, you can help each other through the program.
Barbara Porter: And listen, there's always gonna be things that are difficult in the online programs, the professors, they'll have out office hours, they'll have support there for you if you need it. So don't be afraid to take advantage of that support, but also there's so much out there on the internet. So if you have a question about something and you need a quick answer, Google's usually pretty good and now ChatGPT is usually pretty good at helping you find a quick answer. And these programs are shifting to that.
Barbara Porter: Everyone's able to use ChatGPT, everyone's able to use Google. So it's really understanding the why and the correct way to use the tools. That's what this program will teach you, which gives you the skillset that separates you from somebody who's just Googling stuff, looking for an answer.
Aisha Rudd: Great answers. Thank you so much for that. And great question also, Alicia, thank you for typing that in for us to discuss. Looks like we have another question. Pima, welcome. Pima's question is, “What would you suggest a next educational goal be after a master's in data science?”
Barbara Porter: So I'll start first this time.
Aisha Rudd: Sure.
Barbara Porter: It's funny, 'cause I just had this discussion with a machine learning intern I had this summer. And he asked me exactly that. Should he consider a PhD or should he consider going for an MBA? And I said to him, I said, "What kind of desk do you see yourself sitting behind in 15 years? Do you wanna be an executive managing people and managing processes and guiding a company at a higher level? Or do you really wanna be an extreme specialist in AI, machine learning, things like that?" And the answer is different for everybody. You really have to think about what kind of work you enjoy doing and what kind of work you think you're gonna enjoy doing 10, 15, 20 years from now.
Barbara Porter: But I think those, if you like data science and you feel that it may be a good option for you, next in my mind, the next two possible education goals, having that MBA and having that perspective on business can make you very powerful. Having that data strength and background and then the business knowledge can make you very powerful. But if you're less interested about how to run a business, but you really wanna focus on being a specialist in doing stuff with data that's going to propel a business and help a business evolve, you may consider a more advanced degree in tech and PhD or a DEng or something like that.
Aisha Rudd: Thank you so much Barbara. Sanyogita, did you have anything to add to that question?
Sanyogita Shamsunder: Yeah, this is a question that I get asked a lot and sometimes I also give unsolicited advice to my children and their friends and so on. So for me, it is what interests you, right? I mean, I have a PhD, I have done deep technical work, but I've also branched off into doing something. So it all depends on what excites you. I don't, I mean, there are not many jobs that require a PhD, but then if you get a PhD, you're not necessarily closed off from other jobs. So if you're interested in learning a particular area to an extent and to be able to take your analytical skills to the next level, then maybe you want to do a PhD. I mean, if you'd like to go to school, learn more, it all depends on your individual interests. So you should ask yourselves, what is it?
Sanyogita Shamsunder: Don't necessarily do it just because you get the next job, but be interested in it, like do it because you're interested. And I think that's what will keep you going because everything, things are changing so much. I mean, my job didn't exist 20, if you asked me when I was in college, what would I be doing 20 years from now? I am like, "I have no idea" Right? It's every day there are new things that are coming up. So I feel like you go to school to learn to learn. That's the most important thing. How do you learn to learn new things? That should be your goal. And if you're interested in learning more in a particular area and you want to be a researcher, that's fine. Or if you want to, you learn, but you still want to expand out, that's fine too. So it all depends. That's a difficult question, it's very individual. No two career trajectories are same.
Aisha Rudd: Well, I think you both answered it very well. Thank you so much, Sanyogita and also Barbara. And then we have another question from Francis. We're going to make this our last question for tonight, for time. But Francis has a great question for us. “The most significant change facing the tech world is the advancement of AI. And it's clear that as this technology continues to develop, more and more tech jobs will be replaced by AI. My question is, while we are in this data science program, what data or programming skills should we learn on the side via DataCamp, Coursera, or YouTube to be ahead of this emerging technology?” Great question, Francis, to close this out. And I'll open that up to you both whoever wants to take that one.
Barbara Porter: Yeah, so listen, you're gonna learn a lot of the tools and skills that you need as a part of this program. Certainly, if you have not programmed in Python already, I would recommend looking into that starting tonight. Go Google it, how to learn Python, watch some videos, things like that. Just get a feel for it. It's not hard. I think you should have a good feel for statistics. And there are a lot of machine learning tools out there like Scikit that I'm sure you will get exposure to during this program.
Barbara Porter: In my company, we used to use Tableau for a lot of data analysis, and we've since migrated over to Microsoft Power BI. So you could choose to learn about those. I'm not sure if those are tools that they teach you about in the program, but I wouldn't worry so much about the tools, but the concepts you want to get, you're gonna get a good foundation of the concepts in the program, but any that you feel that you're interested in learning more about, or maybe before you even start the program that you want to just make sure you're up to speed, maybe you haven't taken statistics in 20 years or something like that, look on Coursera. There's a lot of free courses. There's a lot of courses, videos on YouTube that can be very helpful.
Aisha Rudd: Thank you so much for that. Sanyogita did you have anything you would like to add to that?
Sanyogita Shamsunder: No, I think Barbara covered it. I mean, look at where the gaps are in your background and focus on that to fill in the gaps, whether it be in your domain or as part of the data science curriculum, if you're programming, for example, if you're weak, then you might want to take some of that, some classes, do some projects on the side and try to understand where the industry is going in your spare time, like watching YouTube. For example, if you're going into the marketing field background with a data science degree, understand what are some things that are most desirable to learn. So fill in those gaps. So look at it that way.
Aisha Rudd: Thank you. Thank you. And I don't know, probably say this for Jeffrey as well, but I appreciate you both, Barbara, Sanyogita. This has been an awesome presentation. Thank you so much for giving us your time this evening to field questions about this growing field. I've learned a lot, taken a lot of great notes that I can share with future candidates. And for anyone that is in the presentation with us now live, here is my contact information along with Jeffrey's as well. Our email address is there. Please feel free to schedule some time, schedule an appointment. I would definitely enjoy connecting with you and getting you moving forward with your educational goals with a master's in data science online through New York Tech. So definitely have enjoyed this. Please take this information down. If you are interested or have questions, it will be my pleasure to discuss this further with you. And I think that is pretty much it. Jeffrey, did you have anything else that you would like to add?
Jeffrey Bania: No, Aisha, I think that very much sums it up. Thank you everyone so, so much for spending time with us this evening. I know it's 7:00 Eastern. So wanna let Barbara get back to her evening. Sanyogita, I know you're on the West Coast. So you're kind of behind there a little bit and have probably some more work day to get through.
Sanyogita Shamsunder: Right.
[laughter]
Jeffrey Bania: But, yes, very much appreciate your precious, precious time. Everyone, thank you so much for being here and don't hesitate to reach out to myself or Aisha should you need anything. Thank you so much.
Barbara Porter: Thank you. What a pleasure. Honored to be with Barbara. Thank you. Bye.
Aisha Rudd: Yes, thank you. Have a great evening.
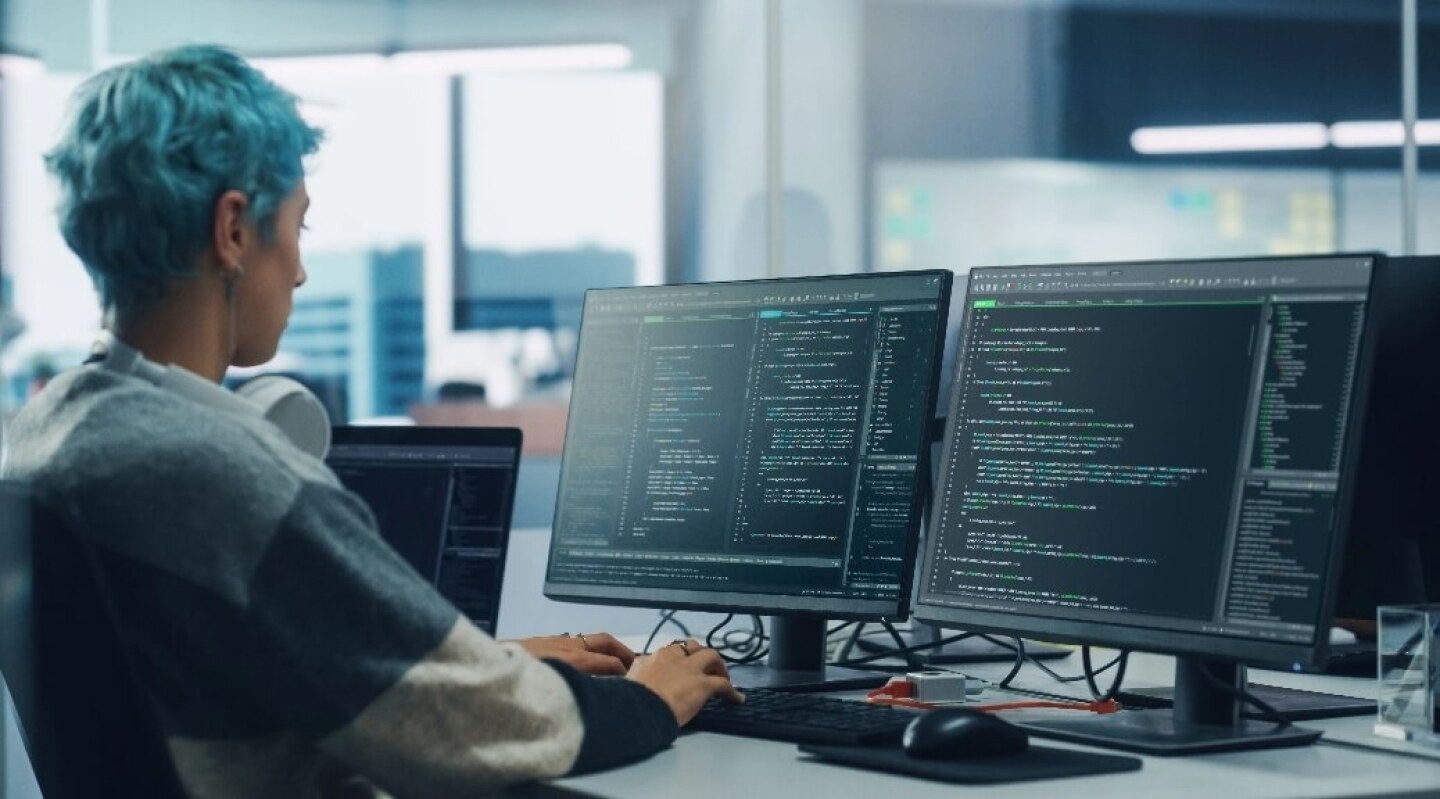
Course Spotlight
DTSC 710: Machine Learning
In this course, students will learn important machine learning (ML) and data mining concepts and algorithms. Emphasis is on basic ideas and intuitions behind ML methods and their applications in activity recognition, and anomaly detection. This course will cover core ML topics such as classification, clustering, feature selection, Bayesian networks, and feature extraction.
Online Master’s in Data Science Faculty
Faculty Spotlights
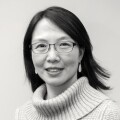
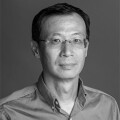
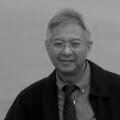
High Growth, Higher Rewards
The demand for data science professionals has exploded in the past decade. As more businesses invest in digital transformation, they’re collecting “oceans of data” that they aren’t equipped to handle but are desperate to capitalize on.5 That means that job opportunities for data scientists and analysts will continue to multiply, both in terms of numbers and compensation.
Employment of data scientists is projected to grow 35% from 2021 to 2031, much faster than the average for all occupations.4 With an average salary of about $120,000, a booming job market, and impressively high job satisfaction, ‘data scientist’ was ranked the third best job in America in 2022.3,6
What can I do with an Online Master’s in Data Science?
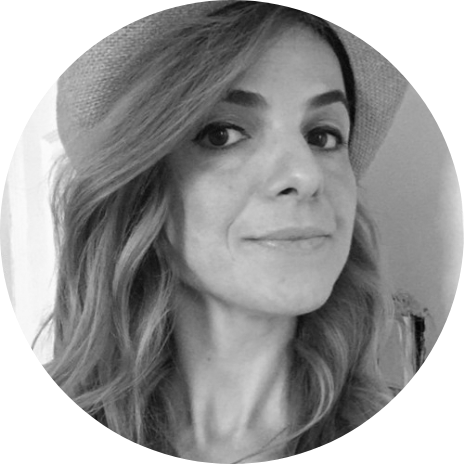
“With data, things that seemed impossible years ago can make life easier today in a very short time. With COVID-19, bipolar disorders, weather forecasts, and so many other uses, data science operations do the impossible.”
Sibel S.
Data Science, M.S. ‘22
Admissions At A Glance
Online Master’s in Data Science requirements:
- Completed application form
- A bachelor’s degree from an accredited institution, with a GPA of 2.85 or higher on a 4.0 scale*
- Copies of undergraduate transcripts
- Copy of college diploma or proof of degree
*If you do not satisfy this criterion, you may still be admitted at the dean's discretion. Talk to an admissions outreach advisor to explore your options.
Frequently Asked Questions
What is an Online Master’s in Data Science?
Download our Program Brochure for more information.
What’s the difference between data science and data analytics?
Data science is a broader field (that actually can encompass data analytics) which requires a knowledge of math, advanced statistics, predictive modeling, machine learning, and programming. It is multi-disciplinary and focuses on making connections, predicting outcomes, and uncovering new hypotheses within and between datasets. Those who earn a master’s in data science are poised to become successful as an applications architect, business intelligence developer, machine learning engineer, or statistician.
Data analytics, on the other hand, involves a closer, usually historical view of data to derive insights and inform business decisions. If you want to earn a master’s degree in data analytics, you should have a strong background in statistics, databases, and modeling. With these skills, you’ll excel as a senior data analyst, database administrator, or consultant.
Is a Master’s in Data Science worth it?
With this designation, as well as the valuable New York network and career resources that New York Tech provides, you’ll find that the Online Data Science, M.S. is well worth your time. So much so, in fact, that New York Tech is ranked No. 9 in New York and in the top 10% in the U.S. for Return on Investment (ROI)14, 2 As one of the top 25 universities in the region, we pride ourselves on putting our students and their needs first.1
Admissions Deadlines
Connect With Us
Stay Up To Date
Read the latest articles on data science topics, trends, and technologies.
Find Job Opportunities
Explore career options and average salaries in data science.
- Retrieved on March 4, 2024, from usnews.com/best-colleges/rankings/regional-universities-north?_sort=rank&_sortDirection=asc
- Retrieved on March 4, 2024, from cew.georgetown.edu/cew-reports/collegeroi/
- Retrieved on March 4, 2024, from glassdoor.com/List/Best-Jobs-in-America-LST_KQ0,20.htm
- Retrieved on March 4, 2024, from bls.gov/ooh/math/data-scientists.htm
- Retrieved on March 4, 2024, from fortune.com/education/business/articles/2022/01/19/how-much-do-grads-with-a-data-science-degree-make/
- Retrieved on March 4, 2024, from indeed.com/career/data-analyst/salaries
- Retrieved on March 4, 2024, from indeed.com/career/database-administrator/salaries
- Retrieved on March 4, 2024, from indeed.com/career/business-analyst/salaries
- Retrieved on March 4, 2024, from glassdoor.com/Salaries/cyber-security-engineer-salary-SRCH_KO0,23.htm
- Retrieved on March 4, 2024, from glassdoor.com/Salaries/it-director-salary-SRCH_KO0,11.htm
- Retrieved on March 4, 2024, from ziprecruiter.com/Salaries/SQL-Developer-Salary
- Retrieved on March 4, 2024, from indeed.com/career/machine-learning-engineer/salaries
- Retrieved on March 4, 2024, from glassdoor.com/Salaries/solutions-architect-salary-SRCH_KO0,19.htm
- Retrieved on March 4, 2023, from cew.georgetown.edu/cew-reports/lowincome/